NATURE
27 November 2003
Home | Destpêk | Ana Sayfa
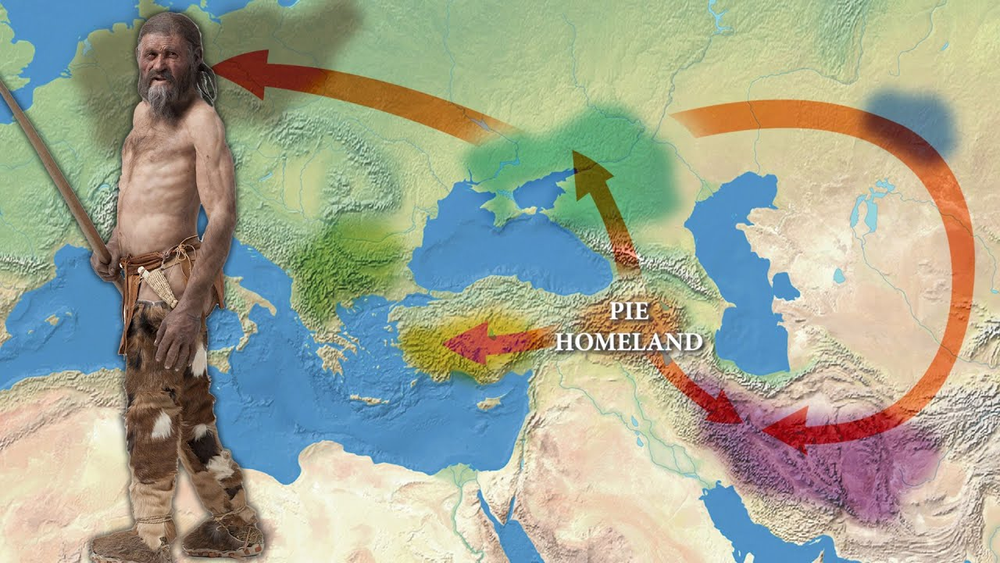
NEW DNA ANALYSIS SHED LIGHT TO INDO-EUROPEAN HOMELAND
Humanity first spread from Zagros to Europe and South Asia
Could this all be a coincidence?
-- There is a Britan (Beritan/Bêrtî) Kurdish tribe (Serhed)
-- There is a German (Germian) Kurdish tribe (Kerkuk, Kelar)
-- Goti (Goyi) kurd tribe (Hekari)
-- There is the Swedî (Svedki) Kurdish tribe (Çolig, Bingol)
-- We do not know by now if there is a name and place in Kurdistan which is connected with the Ions (Greeks), but if it is researched, it definitely exists.
In the Research on the Origin of Indo-European Languages, initiated by the University of Auckland in New Zealand in 1980 and partially published in the prestigious journal Nature in the autumn of 2003, "the origin of all Indo-European languages" was found in the Neolithic Period of Kurdistan. It was claimed to be the revir basin of the Tigris and Eufrat rivers.
Probably this is not just a coincidence.
When the origins of Hindu-European languages ??and tribes were investigated at Auckland University, there was no Kurdish-speaking etymologist in this study group. Today's Kurdish etymologists quite rightly find the work of this group very incomplete. Because when the information about Kurdish, which is a very old Indo-European language, is considered in this group, only the missing information about the Kurdish language given by 17th and 18th century European archaeologists, travelers and orientalists has been evaluated. It has been revealed today that the approaches of these archaeologists and travelers regarding the Kurds and the Kurdish language are centered on racism and Christian chauvinism.
Now there are Kurdish etymologists who speak Greek and also speak very good ancient Greek, and they reveal that the ancient Greek language shows great similarities with the Kurdish language, which is a much older language than Greek. One of these Kurdish etymologists is Ali Karduxos.
Do you think this is just a coincidence?
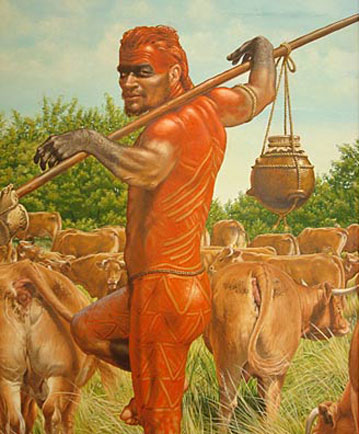
Prehistoric Farmer of Eastern Anatolia (Kurdistan)
"The Kurds are the grandchildren of the first shepherds who have been in the mountains of Kurdistan since the Stone Age."
Geneticists David Comas
Nature 426, 435 - 439 (27 November 2003); doi:10.1038/nature02029
Language-tree divergence times support the Anatolian theory of
Indo-European origin
RUSSELL D. GRAY AND QUENTIN D. ATKINSON
Department of Psychology, University of Auckland, Private Bag 92019,
Auckland 1020, New Zealand
Correspondence and requests for materials should be addressed to R.G.
(rd.gray@auckland.ac.nz).
Languages, like genes, provide vital clues about human history1, 2.
The origin of the Indo-European language family is "the most
intensively studied, yet still most recalcitrant, problem of historical
linguistics"3. Numerous genetic studies of Indo-European origins
have also produced inconclusive results4-6. Here we analyse linguistic
data using computational methods derived from evolutionary biology.
We test two theories of Indo-European origin: the 'Kurgan expansion'
and the 'Anatolian farming' hypotheses. The Kurgan theory centres
on possible archaeological evidence for an expansion into Europe and
the Near East by Kurgan horsemen beginning in the sixth millennium
BP7, 8. In contrast, the Anatolian theory claims that Indo-European
languages expanded with the spread of agriculture from Anatolia around
8,000–9,500 years BP9. In striking agreement with the Anatolian
hypothesis, our analysis of a matrix of 87 languages with 2,449 lexical
items produced an estimated age range for the initial Indo-European
divergence of between 7,800 and 9,800 years BP. These results were
robust to changes in coding procedures, calibration points, rooting
of the trees and priors in the bayesian analysis.
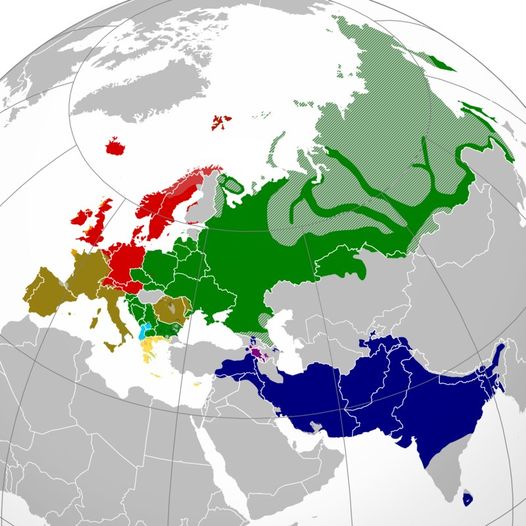
Map of Indo-European Geography
Historical linguists traditionally use the 'comparative method' to
construct language family trees from discrete lexical, morphological
and phonological data. Unfortunately, although the comparative method
can provide a relative chronology, it cannot provide absolute date
estimates. An alternative method of analysis is glottochronology.
This derivative of lexicostatistics is a distance-based approach to
language-tree construction that enables absolute dates to be estimated10.
Glottochronology uses the percentage of shared 'cognates' between
languages to calculate divergence times by assuming a constant rate
of lexical replacement or 'glottoclock'. Cognates are words inferred
to have a common historical origin because of systematic sound correspondences
and clear similarities in form and meaning. Despite some initial enthusiasm,
the method has been heavily criticized and is now largely discredited11,
12. Criticisms of glottochronology, and distance-based methods in
general, tend to fall into four main categories: first, by summarizing
cognate data into percentage scores, much of the information in the
discrete character data is lost, greatly reducing the power of the
method to reconstruct evolutionary history accurately13; second, the
clustering methods used tend to produce inaccurate trees when lineages
evolve at different rates, grouping together languages that evolve
slowly rather than languages that share a recent common ancestor12,
14; third, substantial borrowing of lexical items between languages
makes tree-based methods inappropriate; and fourth, the assumption
of a strict glottoclock rarely holds, making date estimates unreliable11.
For these reasons, historical linguists have generally abandoned efforts
to estimate absolute ages. Dixon15 epitomizes this view with his assertion
that, on the basis of linguistic data, the age of Indo-European "could
be anything—4,000 years BP or 40,000 years BP are both perfectly
possible (as is any date in between)".
Recent advances in computational phylogenetic methods, however, provide
possible solutions to the four main problems faced by glottochronology.
First, the problem of information loss that comes from converting
discrete characters into distances can be overcome by analysing the
discrete characters themselves to find the optimal tree(s). Second,
the accuracy of tree topology and branch-length estimation can be
improved by using models of evolution. Maximum-likelihood methods
generally outperform distance and parsimony approaches in situations
where there are unequal rates of change14. Moreover, uncertainty in
the estimation of tree topology, branch lengths and parameters of
the evolutionary model can be estimated using bayesian Markov chain
Monte Carlo16 (MCMC) methods in which the frequency distribution of
the sample approximates the posterior probability distribution of
the trees17. All subsequent analyses can then incorporate this uncertainty.
Third, lexical items that are obvious borrowings can be removed from
the analysis, and computational methods such as split decomposition18,
which do not force the data to fit a tree model, can be used to check
for non-tree-like signals in the data. Finally, the assumption of
a strict clock can be relaxed by using rate-smoothing algorithms to
model rate variation across the tree. The penalized-likelihood19 model
allows rate variation between lineages while incorporating a 'roughness
penalty' that penalizes changes in rate from branch to branch. This
smoothes inferred rate variation across the tree so that the age of
any node can be estimated even under conditions of rate heterogeneity.
We applied likelihood models of lexical evolution, bayesian inference
of phylogeny and rate-smoothing algorithms to a matrix of 87 Indo-European
languages with 2,449 cognate sets coded as discrete binary characters.
This coding was based on the Indo-European database of Dyen et al.20,
with the addition of three extinct languages. Examining subsets of
languages using split decomposition revealed a strong tree-like signal
in the data, and a preliminary parsimony analysis produced a consistency
index of 0.48 and a retention index of 0.76, well above what would
be expected from biological data sets of a similar size21. The consensus
tree from an initial analysis is shown in Fig. 1a. The topology of
the tree is consistent with the traditional Indo-European language
groups22. All of these groups are monophyletic and supported by high
posterior probability values. Recent parsimony and compatibility analyses
have also supported these groupings, as well as a Romano-Germano-Celtic
supergroup, the early divergence of Greek and Armenian lineages23,
and the basal position of Tocharian24. The consensus tree also reflects
traditional uncertainties in the relationships between the major Indo-European
language groups. For instance, historical linguists have not resolved
the position of the Albanian group and our results clearly reflect
this uncertainty (the posterior probability of the Albanian/Indo-Iranian
group is only 0.36).
Figure 1 Consensus tree and divergence-time estimates. Full legend
High resolution image and legend (154k)One important advantage of
the bayesian MCMC approach is that any inferences are not contingent
on a specific tree topology. Trees are sampled in proportion to their
posterior probability, providing a direct measure of uncertainty in
the tree topology and branch-length estimates. By estimating divergence
times across the MCMC sample distribution of trees, we can explicitly
account for variability in the age estimates due to phylogenetic uncertainty,
and hence calculate a confidence interval for the age of any node.
We estimated divergence times by constraining the age of 14 nodes
on each tree in accordance with historically attested events (see
Supplementary Information). We then used penalized-likelihood rate
smoothing to calculate divergence times without the assumption of
rate constancy19. Another advantage of the bayesian framework is that
prior knowledge of language relationships can be incorporated into
the analysis. To ensure that the sample was consistent with well-established
linguistic relationships, we filtered the 10,000-tree sample using
a constraint tree (Fig. 1b). We used the resulting distribution of
3,500 estimates of basal divergence times to create a confidence interval
for the age of the Indo-European language family (Fig. 1b).
A key part of any bayesian phylogenetic analysis is an assessment
of the robustness of the inferences. One important potential cause
of error is cognacy judgements. In the initial analysis, we included
all cognate sets in the Dyen et al. database20 in an effort to maximize
phylogenetic signal. To assess the impact of different levels of stringency
in the cognacy judgements, we repeated the analysis after removing
all cognate sets identified by Dyen et al. as 'doubtful'. 'Doubtful
cognates' (for instance, possible chance similarities) could falsely
increase similarities between languages and thus lead to an underestimate
of the divergence times. Unrecognized borrowing between closely related
languages would have a similar effect. Conversely, borrowing between
distantly related languages will falsely inflate branch lengths at
the base of the tree and thus increase divergence-time estimates.
With the doubtful cognates removed, the conservative coding led to
a similar estimate of Indo-European language relationships to that
produced using the original coding. The relationships within each
of the 11 main groups were unchanged. Only the placement of the weakly
supported basal branches differed (Fig. 1c). More significantly, the
divergence-time estimates increased, suggesting that the effects of
chance similarities and unrecognized borrowings between closely related
languages might have outweighed those of borrowings between distantly
related languages. In other words, our initial analysis is likely
to have underestimated the age of Indo-European.
The constraint tree used to filter the MCMC sample of trees also contained
assumptions about Indo-European history that might have biased the
results. We therefore repeated the analyses using a more relaxed set
of constraints (Fig. 1d). This produced a divergence-time distribution
and consensus tree almost identical to the original sample distribution
(Fig. 1d).
Another potential bias lay in the initial coding procedure, which
made no allowance for missing cognate information. The languages at
the base of the tree (Hittite, Tocharian A and Tocharian B) may appear
to lack cognates found in other languages because our knowledge of
these extinct languages is limited to reconstructions from ancient
texts. This uneven sampling might have increased basal branch lengths
and thus inflated estimates of divergence times. We tested this possibility
by recoding apparently absent cognates as uncertainties (absent or
present) and re-running the analyses. Although divergence-time estimates
decreased slightly, the effect was only small (Fig. 1e).
Finally, although there is considerable support for Hittite (an extinct
Anatolian language) as the most appropriate root for Indo-European22,
23, rooting the tree with Hittite could be claimed to bias the analysis
in favour of the Anatolian hypothesis. We thus re-ran the analysis
using the consensus tree in Fig. 1 rooted with Balto-Slavic, Greek
and Indo-Iranian as outgroups. This increased the estimated divergence
time from 8,700 years BP to 9,600, 9,400 and 10,100 years BP, respectively.
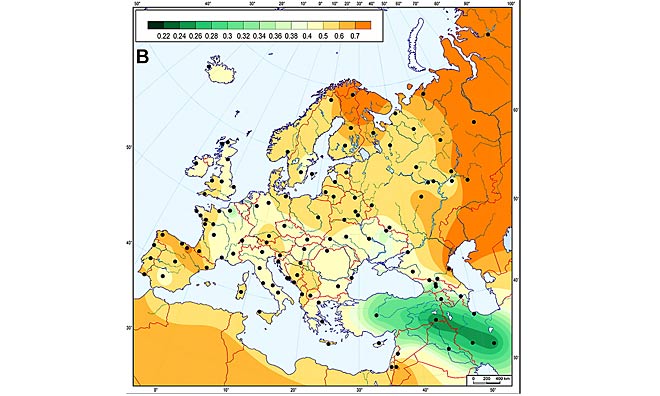
The pattern and timing of expansion suggested by the four analyses
in Fig. 1 is consistent with the Anatolian farming theory of Indo-European
origin. Radiocarbon analysis of the earliest Neolithic sites across
Europe suggests that agriculture arrived in Greece at some time during
the ninth millennium BP and had reached as far as Scotland by 5,500
years BP25. Figure 1 shows the Hittite lineage diverging from Proto-Indo-European
around 8,700 years BP, perhaps reflecting the initial migration out
of Anatolia. Tocharian, and the Greco-Armenian lineages are shown
as distinct by 7,000 years BP, with all other major groups formed
by 5,000 years BP. This scenario is consistent with recent genetic
studies supporting a Neolithic, Near Eastern contribution to the European
gene pool4, 6. The consensus tree also shows evidence of a period
of rapid divergence giving rise to the Italic, Celtic, Balto-Slavic
and perhaps Indo-Iranian families that is intriguingly close to the
time suggested for a possible Kurgan expansion. Thus, as observed
by Cavalli-Sforza et al.26, these hypotheses need not be mutually
exclusive.
Phylogenetic methods have revolutionized evolutionary biology over
the past 20 years and are now starting to take hold in other areas
of historical inference2, 23, 24, 27-29. The model-based bayesian
framework used in this paper offers several advantages over previous
applications of computational methods to language phylogenies. This
approach allowed us to: identify sections in the language tree that
were poorly supported; explicitly incorporate this uncertainty in
tree typology and branch-length estimates in our analysis; test the
possible effects of borrowing, chance similarities and bayesian priors
on our analysis; and estimate divergence times without the assumption
of a strict glottoclock. The challenge of making accurate inferences
about human history is an extremely demanding one, requiring the integration
of archaeological, genetic, cultural and linguistic data. The combination
of computational phylogenetic methods and lexical data to test archaeological
hypotheses is a step forward in this challenging and fascinating task.
Methods
Data and coding Data were sourced from the comparative Indo-European
database created by Dyen et al.20. The database records word forms
and cognacy judgements in 95 languages across the 200 items in the
Swadesh word list. This list consists of items of basic vocabulary
such as pronouns, numerals and body parts that are known to be relatively
resistant to borrowing. For example, although English is a Germanic
language, it has borrowed around 50% of its total lexicon from French
and Latin. However, only about 5% of English entries in the Swadesh
200-word list are clear Romance language borrowings1. Where borrowings
were obvious, Dyen et al. did not score them as cognate and thus they
were excluded from our analysis; 11 of the speech varieties that were
not coded by Dyen et al. were also excluded. To facilitate reconstruction
of some of the oldest language relationships, we added three extinct
Indo-European languages, thought to fit near the base of the tree
(Hittite, Tocharian A and Tocharian B). Word form and cognacy judgements
for all three languages were made on the basis of multiple sources
to ensure reliability. The presence or absence of words from each
cognate set was coded as '1' or '0', respectively, to produce a binary
matrix of 2,449 cognates in 87 languages.
Tree construction Language trees were constructed using a 'restriction
site' model of evolution that allows unequal character-state frequencies
and gamma-distributed character-specific rate heterogeneity (MrBayes
version 2.01; ref. 30). We used default 'flat' priors for the rate
matrix, branch lengths, gamma shape parameter and site-specific rates.
The results were found to be robust to changes in these priors. For
example, repeating the analyses with an exponential branch-length
prior produced a 95% confidence interval for the basal divergence
time of between 7,100 and 9,200 years BP.
The program was run ten times using four concurrent Markov chains.
Each run generated 1,300,000 trees from a random starting phylogeny.
On the basis of an autocorrelation analysis, only every 10,000th tree
was sampled to ensure that consecutive samples were independent. A
'burn-in' period of 300,000 trees for each run was used to avoid sampling
trees before the run had reached convergence. Log-likelihood plots
and an examination of the post-burn-in tree topologies showed that
the runs had indeed reached convergence by this time. For each analysis
a total of 1,000 trees were sampled and rooted with Hittite. The branch
between Hittite and the rest of the tree was split at the root such
that half its length was assigned to the Hittite branch and half to
the remainder of the tree; divergence-time estimates were found to
be robust to threefold alterations of this allocation.
Divergence-time estimates Eleven nodes corresponding to the points
of initial divergence in all of the major language subfamilies were
given minimum and/or maximum ages on the basis of known historical
information (see Supplementary Information). The ages of all terminal
nodes on the tree, representing languages spoken today, were set to
zero by default. Hittite and the Tocharic languages were constrained
in accordance with estimated ages of the source texts. Relatively
broad date ranges were chosen to avoid making disputable, a priori
assumptions about Indo-European history. A likelihood ratio test with
the extinct languages removed revealed that rates were significantly
non-clock-like ( 2 = 787.3, d.f. = 82, P < 0.001). Divergence-time
estimates were thus made using the semi-parametric, penalized-likelihood
model of rate variation implemented in R8s (version 1.50)19. The cross-validation
procedure was applied to the majority-rule consensus tree (Fig. 1)
to determine the optimal value of the rate-smoothing parameter. Step-by-step
removal of each of the 14 age constraints on the consensus tree revealed
that divergence-time estimates were robust to calibration errors.
For 13 nodes, the reconstructed age was within 390 years of the original
constraint range. Only the reconstructed age for Hittite showed an
appreciable variation from the constraint range. This may be attributable
to the effect of missing data associated with extinct languages. Reconstructed
ages at the base of the tree ranged from 10,400 years BP with the
removal of the Hittite age constraint, to 8,500 years BP with the
removal of the Iranian group age constraint.
Supplementary information accompanies this paper.
Received 18 July 2003; accepted 22 August 2003
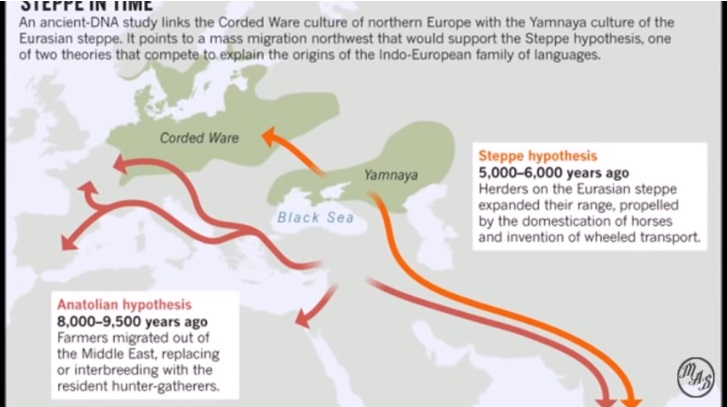
References
1. Pagel, M. in Time Depth in Historical Linguistics (eds Renfrew,
C., McMahon, A. & Trask, L.) 189–207 (The McDonald Institute
for Archaeological Research, Cambridge, UK, 2000)
2. Gray, R. D. & Jordan, F. M. Language trees support the express-train
sequence of Austronesian expansion. Nature 405, 1052–1055 (2000)
| Article | PubMed | ISI | ChemPort |3. Diamond, J. & Bellwood,
P. Farmers and their languages: the first expansions. Science 300,
597–603 (2003) | Article | PubMed | ISI | ChemPort |4. Richards,
M. et al. Tracing European founder lineage in the Near Eastern mtDNA
pool. Am. J. Hum. Genet. 67, 1251–1276 (2000) | PubMed | ISI
| ChemPort |5. Semoni, O. et al. The genetic legacy of Paleolithic
Homo sapiens in extant Europeans: a Y chromosome perspective. Science
290, 1155–1159 (2000) | Article | PubMed | ISI | ChemPort |6.
Chikhi, L., Nichols, R. A., Barbujani, G. & Beaumont, M. A. Y
genetic data support the Neolithic Demic Diffusion Model. Proc. Natl
Acad. Sci. USA 99, 11008–11013 (2002) | Article | PubMed | ChemPort
|7. Gimbutas, M. The beginning of the Bronze Age in Europe and the
Indo-Europeans 3500–2500 B.C. J. Indo-Eur. Stud. 1, 163–214
(1973)
8. Mallory, J. P. Search of the Indo-Europeans: Languages, Archaeology
and Myth (Thames & Hudson, London, 1989)
9. Renfrew, C. in Time Depth in Historical Linguistics (eds Renfrew,
C., McMahon, A. & Trask, L.) 413–439 (The McDonald Institute
for Archaeological Research, Cambridge, UK, 2000)
10. Swadesh, M. Lexico-statistic dating of prehistoric ethnic contacts.
Proc. Am. Phil. Soc. 96, 453–463 (1952)
11. Bergsland, K. & Vogt, H. On the validity of glottochronology.
Curr. Anthropol. 3, 115–153 (1962) | Article | ISI |12. Blust,
R. in Time Depth in Historical Linguistics (eds Renfrew, C., McMahon,
A. & Trask, L.) 311–332 (The McDonald Institute for Archaeological
Research, Cambridge, UK, 2000)
13. Steel, M. A., Hendy, M. D. & Penny, D. Loss of information
in genetic distances. Nature 333, 494–495 (1988) | Article |
PubMed |14. Swofford, D. L., Olsen, G. J., Waddell, P. J. & Hillis,
D. M. in Molecular Systematics (eds Hillis, D., Moritz, C. & Mable,
B. K.) 407–514 (Sinauer Associates, Inc, Sunderland, Massachusetts,
1996)
15. Dixon, R. M. W. The Rise and Fall of Language (Cambridge Univ.
Press, Cambridge, UK, 1997)
16. Metropolis, N., Rosenbluth, A. W., Rosenbluth, M. N., Teller,
A. H. & Teller, E. Equations of state calculations by fast computing
machines. J. Chem. Phys. 21, 1087–1091 (1953) | ISI | ChemPort
|17. Huelsenbeck, J. P., Ronquist, F., Nielsen, R. & Bollback,
J. P. Bayesian inference of phylogeny and its impact on evolutionary
biology. Science 294, 2310–2314 (2001) | Article | PubMed |
ISI | ChemPort |18. Huson, D. H. SplitsTree: analyzing and visualizing
evolutionary data. Bioinformatics 14, 68–73 (1998) | Article
| PubMed | ISI | ChemPort |19. Sanderson, M. R8s, Analysis of Rates
of Evolution,Version 1.50 (Univ. California, Davis, 2002)
20. Dyen, I., Kruskal, J. B. & Black, P. FILE IE-DATA1. Available
at http://www.ntu.edu.au/education/langs/ielex/IE-DATA1 (1997).21.
Sanderson, M. J. & Donoghue, M. J. Patterns of variation in levels
of homoplasy. Evolution 43, 1781–1795 (1989) | ISI |22. Gamkrelidze,
T. V. & Ivanov, V. V. Trends in Linguistics 80: Indo-European
and the Indo-Europeans (Mouton de Gruyter, Berlin, 1995)
23. Rexova, K., Frynta, D. & Zrzavy, J. Cladistic analysis of
languages: Indo-European classification based on lexicostatistical
data. Cladistics 19, 120–127 (2003) | Article | ISI |24. Ringe,
D., Warnow, T. & Taylor, A. IndoEuropean and computational cladistics.
Trans. Philol. Soc. 100, 59–129 (2002) | Article | ISI |25.
Gkiasta, M., Russell, T., Shennan, S. & Steele, J. Neolithic transition
in Europe: the radiocarbon record revisited. Antiquity 77, 45–62
(2003) | ISI |26. Cavalli-Sforza, L. L., Menozzi, P. & Piazza,
A. The History and Geography of Human Genes (Princeton Univ. Press,
Princeton, 1994)
27. Holden, C. J. Bantu language trees reflect the spread of farming
across sub-Saharan Africa: a maximum-parsimony analysis. Proc. R.
Soc. Lond. B 269, 793–799 (2002) | Article | PubMed | ISI |28.
Barbrook, A. C., Howe, C. J., Blake, N. & Robinson, P. The phylogeny
of The Canterbury Tales. Nature 394, 839 (1998) | Article | ISI |
ChemPort |29. McMahon, A. & McMahon, R. Finding families: Quantitative
methods in language classification. Trans. Philol. Soc. 101, 7–55
(2003) | Article | ISI |30. Huelsenbeck, J. P. & Ronquist, F.
MRBAYES: Bayesian inference of phylogeny. Bioinformatics 17, 754–755
(2001) | Article | PubMed | ISI | ChemPort |Acknowledgements. We thank
S. Allan, L. Campbell, L. Chikhi, M. Corballis, N. Gavey, S. Greenhill,
J. Hamm, J. Huelsenbeck, G. Nichols, A. Rodrigo, F. Ronquist, M. Sanderson
and S. Shennan for useful advice and/or comments on the manuscript.
Competing interests statement. The authors declare that they have
no competing financial interests.
________________________________________
© 2003 Nature Publishing Group
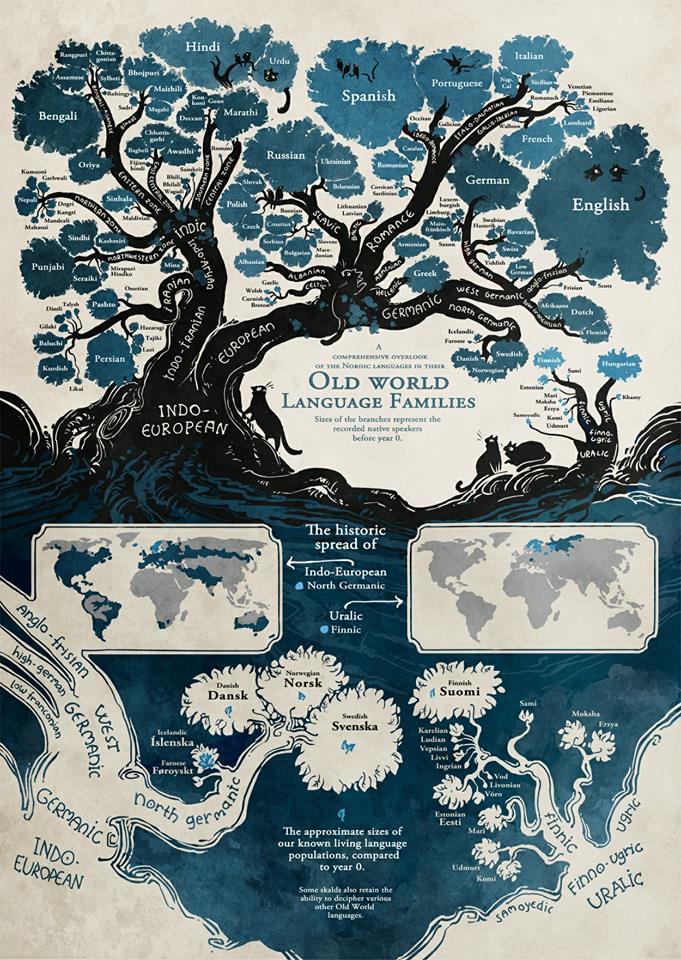
* * *
Language-tree divergence times
support the Anatolian theory of
Indo-European origin
Russell D. Gray and Quentin D. Atkinson
Department of Psychology, University of Auckland, Private Bag 92019,
Auckland 1020, New Zealand
Correspondence to: Russell D. Gray Email: rd.gray@auckland.ac.nz
Languages, like genes, provide vital clues about human history1, 2.
The origin of the Indo-European language family is "the most
intensively studied, yet still most recalcitrant, problem of historical
linguistics"3. Numerous genetic studies of Indo-European origins
have also produced inconclusive results4, 5, 6. Here we analyse linguistic
data using computational methods derived from evolutionary biology.
We test two theories of Indo-European origin: the 'Kurgan expansion'
and the 'Anatolian farming' hypotheses. The Kurgan theory centres
on possible archaeological evidence for an expansion into Europe and
the Near East by Kurgan horsemen beginning in the sixth millennium
BP7, 8. In contrast, the Anatolian theory claims that Indo-European
languages expanded with the spread of agriculture from Anatolia around
8,000–9,500 years bp9. In striking agreement with the Anatolian
hypothesis, our analysis of a matrix of 87 languages with 2,449 lexical
items produced an estimated age range for the initial Indo-European
divergence of between 7,800 and 9,800 years bp. These results were
robust to changes in coding procedures, calibration points, rooting
of the trees and priors in the bayesian analysis.
Historical linguists traditionally use the 'comparative method' to
construct language family trees from discrete lexical, morphological
and phonological data. Unfortunately, although the comparative method
can provide a relative chronology, it cannot provide absolute date
estimates. An alternative method of analysis is glottochronology.
This derivative of lexicostatistics is a distance-based approach to
language-tree construction that enables absolute dates to be estimated10.
Glottochronology uses the percentage of shared 'cognates' between
languages to calculate divergence times by assuming a constant rate
of lexical replacement or 'glottoclock'. Cognates are words inferred
to have a common historical origin because of systematic sound correspondences
and clear similarities in form and meaning. Despite some initial enthusiasm,
the method has been heavily criticized and is now largely discredited11,
12. Criticisms of glottochronology, and distance-based methods in
general, tend to fall into four main categories: first, by summarizing
cognate data into percentage scores, much of the information in the
discrete character data is lost, greatly reducing the power of the
method to reconstruct evolutionary history accurately13; second, the
clustering methods used tend to produce inaccurate trees when lineages
evolve at different rates, grouping together languages that evolve
slowly rather than languages that share a recent common ancestor12,
14; third, substantial borrowing of lexical items between languages
makes tree-based methods inappropriate; and fourth, the assumption
of a strict glottoclock rarely holds, making date estimates unreliable11.
For these reasons, historical linguists have generally abandoned efforts
to estimate absolute ages. Dixon15 epitomizes this view with his assertion
that, on the basis of linguistic data, the age of Indo-European "could
be anything—4,000 years bp or 40,000 years bp are both perfectly
possible (as is any date in between)".
Recent advances in computational phylogenetic methods, however, provide
possible solutions to the four main problems faced by glottochronology.
First, the problem of information loss that comes from converting
discrete characters into distances can be overcome by analysing the
discrete characters themselves to find the optimal tree(s). Second,
the accuracy of tree topology and branch-length estimation can be
improved by using models of evolution. Maximum-likelihood methods
generally outperform distance and parsimony approaches in situations
where there are unequal rates of change14. Moreover, uncertainty in
the estimation of tree topology, branch lengths and parameters of
the evolutionary model can be estimated using bayesian Markov chain
Monte Carlo16 (MCMC) methods in which the frequency distribution of
the sample approximates the posterior probability distribution of
the trees17. All subsequent analyses can then incorporate this uncertainty.
Third, lexical items that are obvious borrowings can be removed from
the analysis, and computational methods such as split decomposition18,
which do not force the data to fit a tree model, can be used to check
for non-tree-like signals in the data. Finally, the assumption of
a strict clock can be relaxed by using rate-smoothing algorithms to
model rate variation across the tree. The penalized-likelihood19 model
allows rate variation between lineages while incorporating a 'roughness
penalty' that penalizes changes in rate from branch to branch. This
smoothes inferred rate variation across the tree so that the age of
any node can be estimated even under conditions of rate heterogeneity.
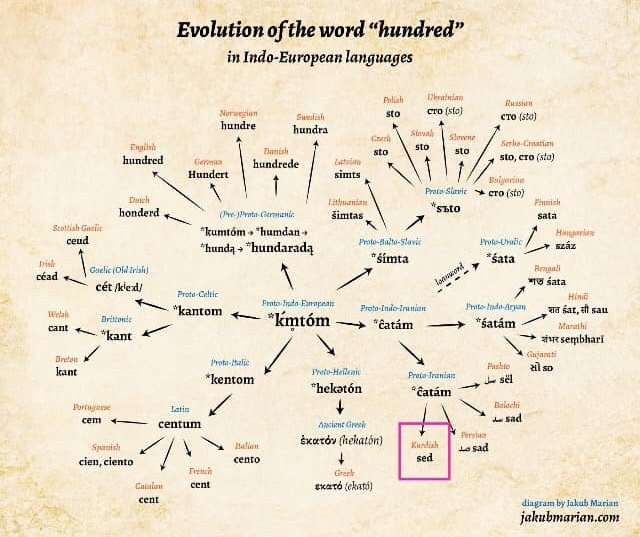
The evolution of the world "hundred" in Indonesia European languages
Kurdish - sed
Russian - ??? (sto)
?Persian - sad
?English - hundred
?German - hundert
We applied likelihood models of lexical evolution, bayesian inference
of phylogeny and rate-smoothing algorithms to a matrix of 87 Indo-European
languages with 2,449 cognate sets coded as discrete binary characters.
This coding was based on the Indo-European database of Dyen et al.20,
with the addition of three extinct languages. Examining subsets of
languages using split decomposition revealed a strong tree-like signal
in the data, and a preliminary parsimony analysis produced a consistency
index of 0.48 and a retention index of 0.76, well above what would
be expected from biological data sets of a similar size21. The consensus
tree from an initial analysis is shown in Fig. 1a. The topology of
the tree is consistent with the traditional Indo-European language
groups22. All of these groups are monophyletic and supported by high
posterior probability values. Recent parsimony and compatibility analyses
have also supported these groupings, as well as a Romano-Germano-Celtic
supergroup, the early divergence of Greek and Armenian lineages23,
and the basal position of Tocharian24. The consensus tree also reflects
traditional uncertainties in the relationships between the major Indo-European
language groups. For instance, historical linguists have not resolved
the position of the Albanian group and our results clearly reflect
this uncertainty (the posterior probability of the Albanian/Indo-Iranian
group is only 0.36).
Figure 1: Consensus tree and divergence-time estimates.
Figure 1 : Consensus tree and divergence-time estimates. Unfortunately
we are unable to provide accessible alternative text for this. If
you require assistance to access this image, or to obtain a text description,
please contact npg@nature.com
a, Majority-rule consensus tree based on the MCMC sample of 1,000
trees. The main language groupings are colour coded. Branch lengths
are proportional to the inferred maximum-likelihood estimates of evolutionary
change per cognate. Values above each branch (in black) express the
bayesian posterior probabilities as a percentage. Values in red show
the inferred ages of nodes in years BP. *Italic also includes the
French/Iberian subgroup. Panels b–e show the distribution of
divergence-time estimates at the root of the Indo-European phylogeny
for: b, initial assumption set using all cognate information and most
stringent constraints (Anatolian, Tocharian, (Greek, Armenian, Albanian,
(Iranian, Indic), (Slavic, Baltic), ((North Germanic, West Germanic),
Italic, Celtic))); c, conservative cognate coding with doubtful cognates
excluded; d, all cognate sets with minimum topological constraints
(Anatolian, Tocharian, (Greek, Armenian, Albanian, (Iranian, Indic),
(Slavic, Baltic), (North Germanic, West Germanic), Italic, Celtic));
e, missing data coding with minimum topological constraints and all
cognate sets. Shaded bars represent the implied age ranges under the
two competing theories of Indo-European origin: blue, Kurgan hypothesis;
green, Anatolian farming hypothesis. The relationship between the
main language groups in the consensus tree for each analysis is also
shown, along with posterior probability values.
High resolution image and legend (154K
One important advantage of the bayesian MCMC approach is that any
inferences are not contingent on a specific tree topology. Trees are
sampled in proportion to their posterior probability, providing a
direct measure of uncertainty in the tree topology and branch-length
estimates. By estimating divergence times across the MCMC sample distribution
of trees, we can explicitly account for variability in the age estimates
due to phylogenetic uncertainty, and hence calculate a confidence
interval for the age of any node. We estimated divergence times by
constraining the age of 14 nodes on each tree in accordance with historically
attested events (see Supplementary Information). We then used penalized-likelihood
rate smoothing to calculate divergence times without the assumption
of rate constancy19. Another advantage of the bayesian framework is
that prior knowledge of language relationships can be incorporated
into the analysis. To ensure that the sample was consistent with well-established
linguistic relationships, we filtered the 10,000-tree sample using
a constraint tree (Fig. 1b). We used the resulting distribution of
3,500 estimates of basal divergence times to create a confidence interval
for the age of the Indo-European language family (Fig. 1b).
A key part of any bayesian phylogenetic analysis is an assessment
of the robustness of the inferences. One important potential cause
of error is cognacy judgements. In the initial analysis, we included
all cognate sets in the Dyen et al. database20 in an effort to maximize
phylogenetic signal. To assess the impact of different levels of stringency
in the cognacy judgements, we repeated the analysis after removing
all cognate sets identified by Dyen et al. as 'doubtful'. 'Doubtful
cognates' (for instance, possible chance similarities) could falsely
increase similarities between languages and thus lead to an underestimate
of the divergence times. Unrecognized borrowing between closely related
languages would have a similar effect. Conversely, borrowing between
distantly related languages will falsely inflate branch lengths at
the base of the tree and thus increase divergence-time estimates.
With the doubtful cognates removed, the conservative coding led to
a similar estimate of Indo-European language relationships to that
produced using the original coding. The relationships within each
of the 11 main groups were unchanged. Only the placement of the weakly
supported basal branches differed (Fig. 1c). More significantly, the
divergence-time estimates increased, suggesting that the effects of
chance similarities and unrecognized borrowings between closely related
languages might have outweighed those of borrowings between distantly
related languages. In other words, our initial analysis is likely
to have underestimated the age of Indo-European.
The constraint tree used to filter the MCMC sample of trees also contained
assumptions about Indo-European history that might have biased the
results. We therefore repeated the analyses using a more relaxed set
of constraints (Fig. 1d). This produced a divergence-time distribution
and consensus tree almost identical to the original sample distribution
(Fig. 1d).
Another potential bias lay in the initial coding procedure, which
made no allowance for missing cognate information. The languages at
the base of the tree (Hittite, Tocharian A and Tocharian B) may appear
to lack cognates found in other languages because our knowledge of
these extinct languages is limited to reconstructions from ancient
texts. This uneven sampling might have increased basal branch lengths
and thus inflated estimates of divergence times. We tested this possibility
by recoding apparently absent cognates as uncertainties (absent or
present) and re-running the analyses. Although divergence-time estimates
decreased slightly, the effect was only small (Fig. 1e).
Finally, although there is considerable support for Hittite (an extinct
Anatolian language) as the most appropriate root for Indo-European22,
23, rooting the tree with Hittite could be claimed to bias the analysis
in favour of the Anatolian hypothesis. We thus re-ran the analysis
using the consensus tree in Fig. 1 rooted with Balto-Slavic, Greek
and Indo-Iranian as outgroups. This increased the estimated divergence
time from 8,700 years bp to 9,600, 9,400 and 10,100 years bp, respectively.
The pattern and timing of expansion suggested by the four analyses
in Fig. 1 is consistent with the Anatolian farming theory of Indo-European
origin. Radiocarbon analysis of the earliest Neolithic sites across
Europe suggests that agriculture arrived in Greece at some time during
the ninth millennium bp and had reached as far as Scotland by 5,500
years bp25. Figure 1 shows the Hittite lineage diverging from Proto-Indo-European
around 8,700 years bp, perhaps reflecting the initial migration out
of Anatolia. Tocharian, and the Greco-Armenian lineages are shown
as distinct by 7,000 years bp, with all other major groups formed
by 5,000 years bp. This scenario is consistent with recent genetic
studies supporting a Neolithic, Near Eastern contribution to the European
gene pool4, 6. The consensus tree also shows evidence of a period
of rapid divergence giving rise to the Italic, Celtic, Balto-Slavic
and perhaps Indo-Iranian families that is intriguingly close to the
time suggested for a possible Kurgan expansion. Thus, as observed
by Cavalli-Sforza et al.26, these hypotheses need not be mutually
exclusive.
Phylogenetic methods have revolutionized evolutionary biology over
the past 20 years and are now starting to take hold in other areas
of historical inference2, 23, 24, 27, 28, 29. The model-based bayesian
framework used in this paper offers several advantages over previous
applications of computational methods to language phylogenies. This
approach allowed us to: identify sections in the language tree that
were poorly supported; explicitly incorporate this uncertainty in
tree typology and branch-length estimates in our analysis; test the
possible effects of borrowing, chance similarities and bayesian priors
on our analysis; and estimate divergence times without the assumption
of a strict glottoclock. The challenge of making accurate inferences
about human history is an extremely demanding one, requiring the integration
of archaeological, genetic, cultural and linguistic data. The combination
of computational phylogenetic methods and lexical data to test archaeological
hypotheses is a step forward in this challenging and fascinating task.
Top of page
Methods
Data and coding
Data were sourced from the comparative Indo-European database created
by Dyen et al.20. The database records word forms and cognacy judgements
in 95 languages across the 200 items in the Swadesh word list. This
list consists of items of basic vocabulary such as pronouns, numerals
and body parts that are known to be relatively resistant to borrowing.
For example, although English is a Germanic language, it has borrowed
around 50% of its total lexicon from French and Latin. However, only
about 5% of English entries in the Swadesh 200-word list are clear
Romance language borrowings1. Where borrowings were obvious, Dyen
et al. did not score them as cognate and thus they were excluded from
our analysis; 11 of the speech varieties that were not coded by Dyen
et al. were also excluded. To facilitate reconstruction of some of
the oldest language relationships, we added three extinct Indo-European
languages, thought to fit near the base of the tree (Hittite, Tocharian
A and Tocharian B). Word form and cognacy judgements for all three
languages were made on the basis of multiple sources to ensure reliability.
The presence or absence of words from each cognate set was coded as
'1' or '0', respectively, to produce a binary matrix of 2,449 cognates
in 87 languages.
Tree construction
Language trees were constructed using a 'restriction site' model of
evolution that allows unequal character-state frequencies and gamma-distributed
character-specific rate heterogeneity (MrBayes version 2.01; ref.
30). We used default 'flat' priors for the rate matrix, branch lengths,
gamma shape parameter and site-specific rates. The results were found
to be robust to changes in these priors. For example, repeating the
analyses with an exponential branch-length prior produced a 95% confidence
interval for the basal divergence time of between 7,100 and 9,200
years BP.
The program was run ten times using four concurrent Markov chains.
Each run generated 1,300,000 trees from a random starting phylogeny.
On the basis of an autocorrelation analysis, only every 10,000th tree
was sampled to ensure that consecutive samples were independent. A
'burn-in' period of 300,000 trees for each run was used to avoid sampling
trees before the run had reached convergence. Log-likelihood plots
and an examination of the post-burn-in tree topologies showed that
the runs had indeed reached convergence by this time. For each analysis
a total of 1,000 trees were sampled and rooted with Hittite. The branch
between Hittite and the rest of the tree was split at the root such
that half its length was assigned to the Hittite branch and half to
the remainder of the tree; divergence-time estimates were found to
be robust to threefold alterations of this allocation.
Divergence-time estimates
Eleven nodes corresponding to the points of initial divergence in
all of the major language subfamilies were given minimum and/or maximum
ages on the basis of known historical information (see Supplementary
Information). The ages of all terminal nodes on the tree, representing
languages spoken today, were set to zero by default. Hittite and the
Tocharic languages were constrained in accordance with estimated ages
of the source texts. Relatively broad date ranges were chosen to avoid
making disputable, a priori assumptions about Indo-European history.
A likelihood ratio test with the extinct languages removed revealed
that rates were significantly non-clock-like (chi2 = 787.3, d.f. =
82, P < 0.001). Divergence-time estimates were thus made using
the semi-parametric, penalized-likelihood model of rate variation
implemented in R8s (version 1.50)19. The cross-validation procedure
was applied to the majority-rule consensus tree (Fig. 1) to determine
the optimal value of the rate-smoothing parameter. Step-by-step removal
of each of the 14 age constraints on the consensus tree revealed that
divergence-time estimates were robust to calibration errors. For 13
nodes, the reconstructed age was within 390 years of the original
constraint range. Only the reconstructed age for Hittite showed an
appreciable variation from the constraint range. This may be attributable
to the effect of missing data associated with extinct languages. Reconstructed
ages at the base of the tree ranged from 10,400 years bp with the
removal of the Hittite age constraint, to 8,500 years bp with the
removal of the Iranian group age constraint.
Pagel, M. in Time Depth in Historical Linguistics (eds Renfrew, C.,
McMahon, A. & Trask, L.) 189-207 (The McDonald Institute for Archaeological
Research, Cambridge, UK, 2000)
Gray, R. D. & Jordan, F. M. Language trees support the express-train
sequence of Austronesian expansion. Nature 405, 1052-1055 (2000) |
Article | PubMed | ISI | ChemPort |
Diamond, J. & Bellwood, P. Farmers and their languages: the first
expansions. Science 300, 597-603 (2003) | Article | PubMed | ISI |
ChemPort |
Richards, M. et al. Tracing European founder lineage in the Near
Eastern mtDNA pool. Am. J. Hum. Genet. 67, 1251-1276 (2000) | PubMed
| ISI | ChemPort |
Semoni, O. et al. The genetic legacy of Paleolithic Homo sapiens
in extant Europeans: a Y chromosome perspective. Science 290, 1155-1159
(2000) | Article | PubMed | ISI | ChemPort |
Chikhi, L., Nichols, R. A., Barbujani, G. & Beaumont, M. A. Y
genetic data support the Neolithic Demic Diffusion Model. Proc. Natl
Acad. Sci. USA 99, 11008-11013 (2002) | Article | PubMed | ChemPort
|
Gimbutas, M. The beginning of the Bronze Age in Europe and the Indo-Europeans
3500-2500 B.C. J. Indo-Eur. Stud. 1, 163-214 (1973)
Mallory, J. P. Search of the Indo-Europeans: Languages, Archaeology
and Myth (Thames & Hudson, London, 1989)
Renfrew, C. in Time Depth in Historical Linguistics (eds Renfrew,
C., McMahon, A. & Trask, L.) 413-439 (The McDonald Institute for
Archaeological Research, Cambridge, UK, 2000)
Swadesh, M. Lexico-statistic dating of prehistoric ethnic contacts.
Proc. Am. Phil. Soc. 96, 453-463 (1952)
Bergsland, K. & Vogt, H. On the validity of glottochronology.
Curr. Anthropol. 3, 115-153 (1962) | Article | ISI |
Blust, R. in Time Depth in Historical Linguistics (eds Renfrew, C.,
McMahon, A. & Trask, L.) 311-332 (The McDonald Institute for Archaeological
Research, Cambridge, UK, 2000)
Steel, M. A., Hendy, M. D. & Penny, D. Loss of information in
genetic distances. Nature 333, 494-495 (1988) | Article | PubMed |
ChemPort |
Swofford, D. L., Olsen, G. J., Waddell, P. J. & Hillis, D. M.
in Molecular Systematics (eds Hillis, D., Moritz, C. & Mable,
B. K.) 407-514 (Sinauer Associates, Inc, Sunderland, Massachusetts,
1996)
Dixon, R. M. W. The Rise and Fall of Language (Cambridge Univ. Press,
Cambridge, UK, 1997)
Metropolis, N., Rosenbluth, A. W., Rosenbluth, M. N., Teller, A.
H. & Teller, E. Equations of state calculations by fast computing
machines. J. Chem. Phys. 21, 1087-1091 (1953) | Article | ISI | ChemPort
|
Huelsenbeck, J. P., Ronquist, F., Nielsen, R. & Bollback, J.
P. Bayesian inference of phylogeny and its impact on evolutionary
biology. Science 294, 2310-2314 (2001) | Article | PubMed | ISI |
ChemPort |
Huson, D. H. SplitsTree: analyzing and visualizing evolutionary data.
Bioinformatics 14, 68-73 (1998) | Article | PubMed | ISI | ChemPort
|
Sanderson, M. R8s, Analysis of Rates of Evolution,Version 1.50 (Univ.
California, Davis, 2002)
Dyen, I., Kruskal, J. B. & Black, P. FILE IE-DATA1. Available
at left fence[www.ntu.edu.au] fence (1997).
Sanderson, M. J. & Donoghue, M. J. Patterns of variation in levels
of homoplasy. Evolution 43, 1781-1795 (1989) | ISI |
Gamkrelidze, T. V. & Ivanov, V. V. Trends in Linguistics 80:
Indo-European and the Indo-Europeans (Mouton de Gruyter, Berlin, 1995)
Rexova, K., Frynta, D. & Zrzavy, J. Cladistic analysis of languages:
Indo-European classification based on lexicostatistical data. Cladistics
19, 120-127 (2003) | Article | ISI |
Ringe, D., Warnow, T. & Taylor, A. IndoEuropean and computational
cladistics. Trans. Philol. Soc. 100, 59-129 (2002) | Article | ISI
|
Gkiasta, M., Russell, T., Shennan, S. & Steele, J. Neolithic
transition in Europe: the radiocarbon record revisited. Antiquity
77, 45-62 (2003) | ISI |
Cavalli-Sforza, L. L., Menozzi, P. & Piazza, A. The History and
Geography of Human Genes (Princeton Univ. Press, Princeton, 1994)
Holden, C. J. Bantu language trees reflect the spread of farming
across sub-Saharan Africa: a maximum-parsimony analysis. Proc. R.
Soc. Lond. B 269, 793-799 (2002) | Article | PubMed | ISI |
Barbrook, A. C., Howe, C. J., Blake, N. & Robinson, P. The phylogeny
of The Canterbury Tales. Nature 394, 839 (1998) | Article | ISI |
ChemPort |
McMahon, A. & McMahon, R. Finding families: Quantitative methods
in language classification. Trans. Philol. Soc. 101, 7-55 (2003) |
Article | ISI |
Huelsenbeck, J. P. & Ronquist, F. MRBAYES: Bayesian inference
of phylogeny. Bioinformatics 17, 754-755 (2001) | Article | PubMed
| ISI | ChemPort | |